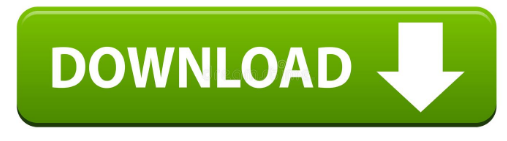
Novelty: The researchers come up with the first text to speech LSTM deep learning model for the Tigrigna language which is critical and will be a baseline for other related research to be done for Tigrigna and other languages. The values of performance, intelligibility, and naturalness are inspiring and show that diphone speech units are good candidates to develop a fully functional speech synthesizer.

Based on the experiment LSTM Deep learning model provides an accuracy of 91.05%, the precision of 78.05%, recall of 86.59 %, and F-score of 83.05% respectively. When it comes to the intelligibility and naturalness of the synthesized speech in the sentence level, it is measured in MOS scale and the overall intelligibility and naturalness of the system are found to be 3.28 and 3.27 respectively. Findings: The overall performance of the system in the word level which is evaluated by NeoSpeech tool is found to be 78% which is fruitful. In this paper LSTM deep learning model was applied to find out accuracy, precision, recall, and Fscore. Tools used in this work are Mathlab, LPC, and python. Methods : The direct observation and review of articles are applied in this research paper to identify the whole strings which are represented the language. Objectives: The purpose of this study is to describe text-to-speech system for the Tigrigna language, using dialog fusion architecture and developing a prototype text-to-speech synthesizer for Tigrigna Language. We also propose enhancements to HEGA that further optimize its performance in this demanding application. This operational requirement on error measurement restricts the application of widely used ANN training algorithms that are based on back propagation of gradients but can be met by our earlier proposed “Heuristically Enhanced Gradient Approximation” (HEGA) algorithm. Thus, the training error needs to be measured after processing the output of the speech synthesizer, instead of measuring it directly at the outputs of the ANN. In this setup, we assume that the training error is obtained by measuring the “perceived distance” between the original (target) and the synthesized speech signals.
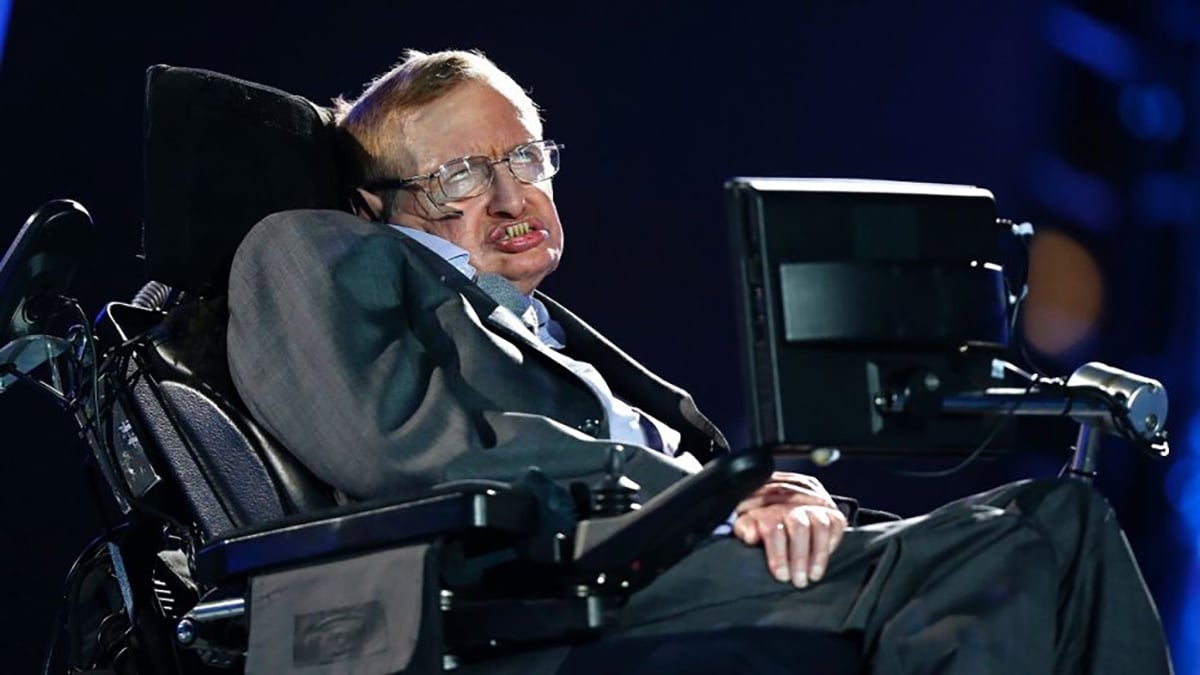
As a technical approximation to such a setup, we use an artificial neural network (ANN) and a speech synthesizer and we study the capability of the ANN to estimate the synthesizer’s parameters targeting desired speech activity. We consider as an example of such an actuator mechanism the human vocal tract where neurons learn to activate its muscles that move the velum, jaw, tongue and the lips, in order to exhibit desired phonetic activity. This work is inspired by the ability of neural systems to control the behavior of specialized actuator mechanisms in living organisms by monitoring the end-effect of their actions.
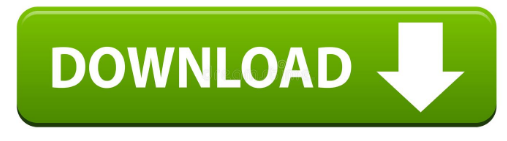